Research
Summary
Our research focuses on three directions: computational neuroscience, neural engineering, integration of data science and neuroscience. All research projects have basic science (what are brain functions and how do neurons represent and transmit information) and translational research (how to help to treat pain or neuropsychiatric disorders) components. Our goal is to develop new tools to tackle important neuroscience questions and invent neurotechnology to improve the brain health or enhance the cognitive / memory functions.
Theme #1: Memory and Sleep
The hippocampus plays an important role in representing space (for spatial navigation) and time (for episodic memory), and is also associated with many brain functions such as learning, emotion and decision-making. Communications between the hippocampus and the cortex underlie memory consolidation that ultimately leads to long-term memory stored in the cortex. Hippocampal impairment or dysfunction affects the performance in learning and working memory. In collaboration with experimental neuroscientists, we use a data-driven approach to explore interesting scientific questions by investigating the ensemble spike activity of the hippocampus and cortex during wakeful behavior and sleep. One specific question of interest is to uncover the hippocampal-neocortical population neuronal representation of memory reactivations (i.e., replay of awake experiences) during SWS (slow wave sleep) and REM (rapid eye movement) sleep. Together, we will integrate multidisciplinary tools such as animal behavior, electrophysiology, optogenetics, Bayesian statistics and neural interfaces to dissect circuit mechanisms of memory and sleep, and their implications to learning and decision-making. (Image Credit: www.bridgemanimages.com)
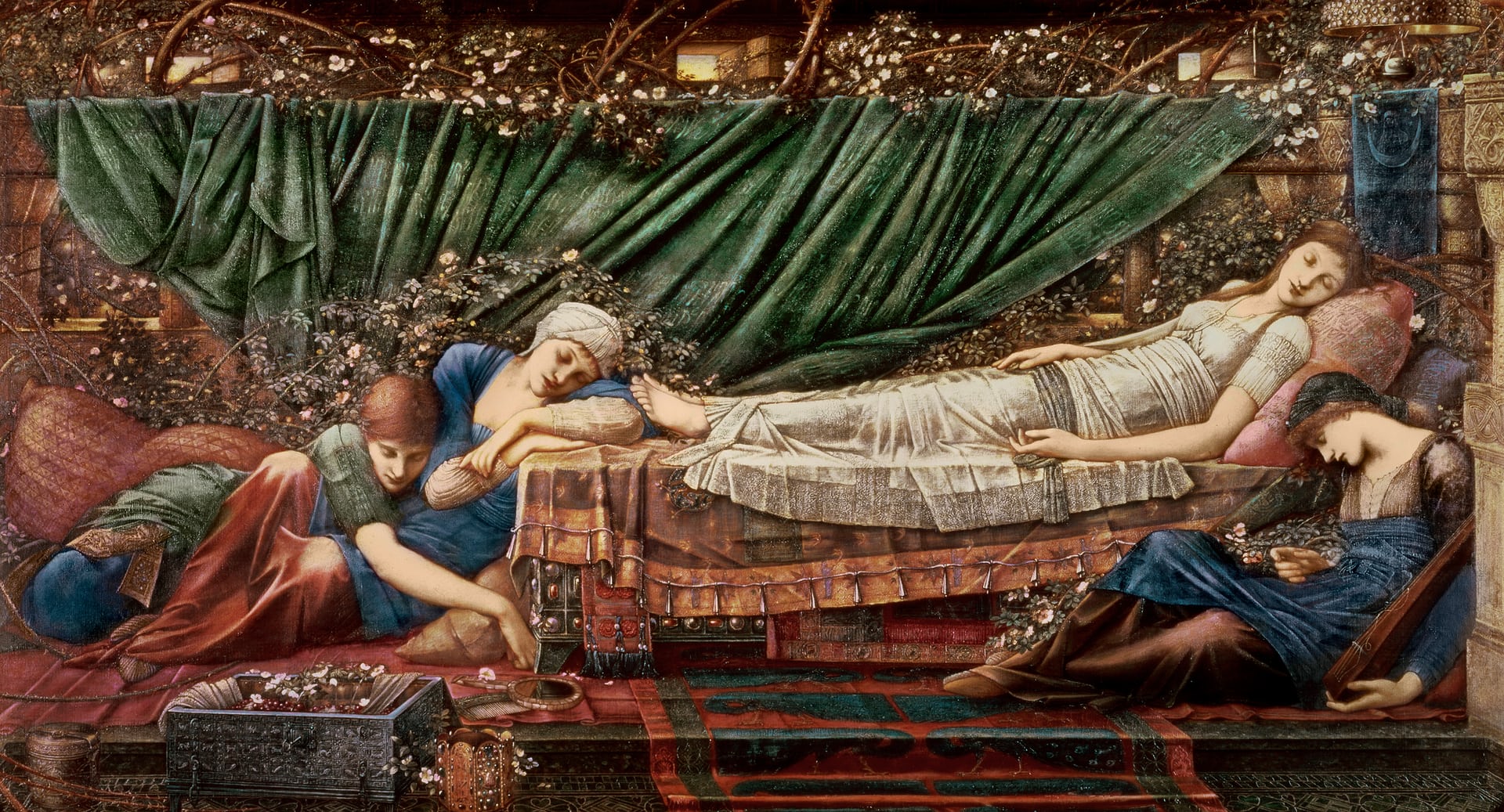
Representative work
Neural Computation, 2014
Neural Computation, 2016
Scientific Reports, 2016
Trends in Neurosciences, 2017
Neural Computation, 2018
Theme #2: Real-time Closed-loop Neural Interfaces
We aim to integrate the knowledge of neurophysiology and engineering tools to conduct closed-loop neuroscience experiments. With the state-of-the-art molecular and genetic engineering tools (e.g., optogenetics) that allow us to probe and manipulate the neural circuits, we design closed-loop neural interfaces to study the link and the causal role of neural circuits to behavior. We are currently investigating two research projects. The first project is to design a closed-loop rodent brain machine interface (BMI) for pain neuromodulation. The BMI consists of a detection arm---which requires us to detect acute pain signals in a timely and precise manner based on in vivo neural recording, and a treatment arm---which employs optogenetic stimulations to activate targeted brain areas to relieve pain behavior. We are also adapting the experimental protocol and shifting the paradigm from animals to human for demand-based pain neuromodulation (e.g., using noninvasive transcranial current stimulation). The second project is to design a real-time BMI system for decoding unsorted hippocampal ensemble spikes during behavior or sleep. To speed up the real-time computation, we employ modern graphic processing unit (GPU) and digital signal processing (DSP) technology. (Image Credit: Getty Images)
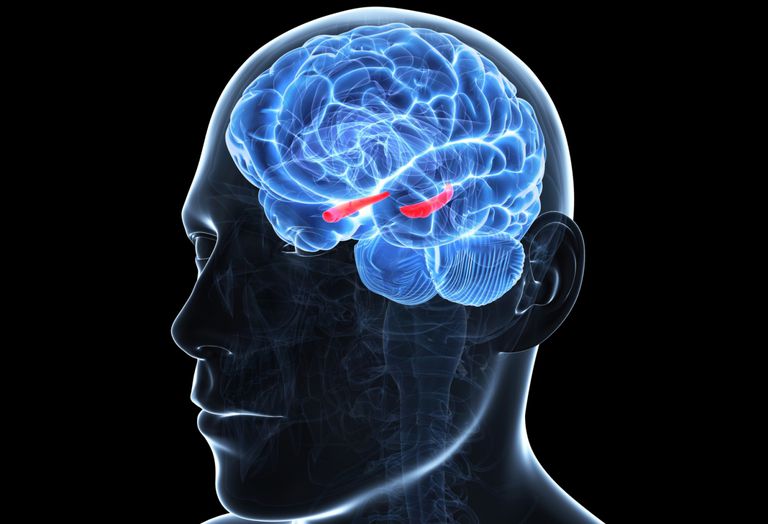
Representative work
Clinical Neurophysiology, 2007
Journal of Neural Engineering, 2017
Journal of Neurophysiology, 2018
Cell Reports, 2018
Journal of Neural Engineering, 2019
Theme #3: Computational Statistics and Machine Learning
Data science has played an increasingly pivotal role in knowledge discovery across many disciplines. Our research has focused on unsupervised learning, with special interests in Bayesian nonparametric methods and deep learning. First, we have developed unsupervised learning methods for Gaussian processes and the hierarchical Dirichlet process-hidden Markov model (HDP-HMM). We have applied these methods to population decoding, dimensionality reduction, data visualization, classification and data mining for neural population spike trains collected from rodents and monkeys during various behavior tasks. We have explored priors in the data structure, such as sparsity and smoothness. Second, motivated by the strengths of discriminative models and deep learning methods, we are currently developing hierarchical hybrid (generative/discriminative) models for analyzing neural population spikes, such as uncovering latent structures of population codes or characterizing nonlinear associations of multiple brain-region population codes. The idea is to overcome the limitation of representation capability in the probabilistic generative model and to capture the complex statistical dependency in spatiotemporal neural patterns. The methodology developed here is universal, and can be extended to other research domains beyond neuroscience.
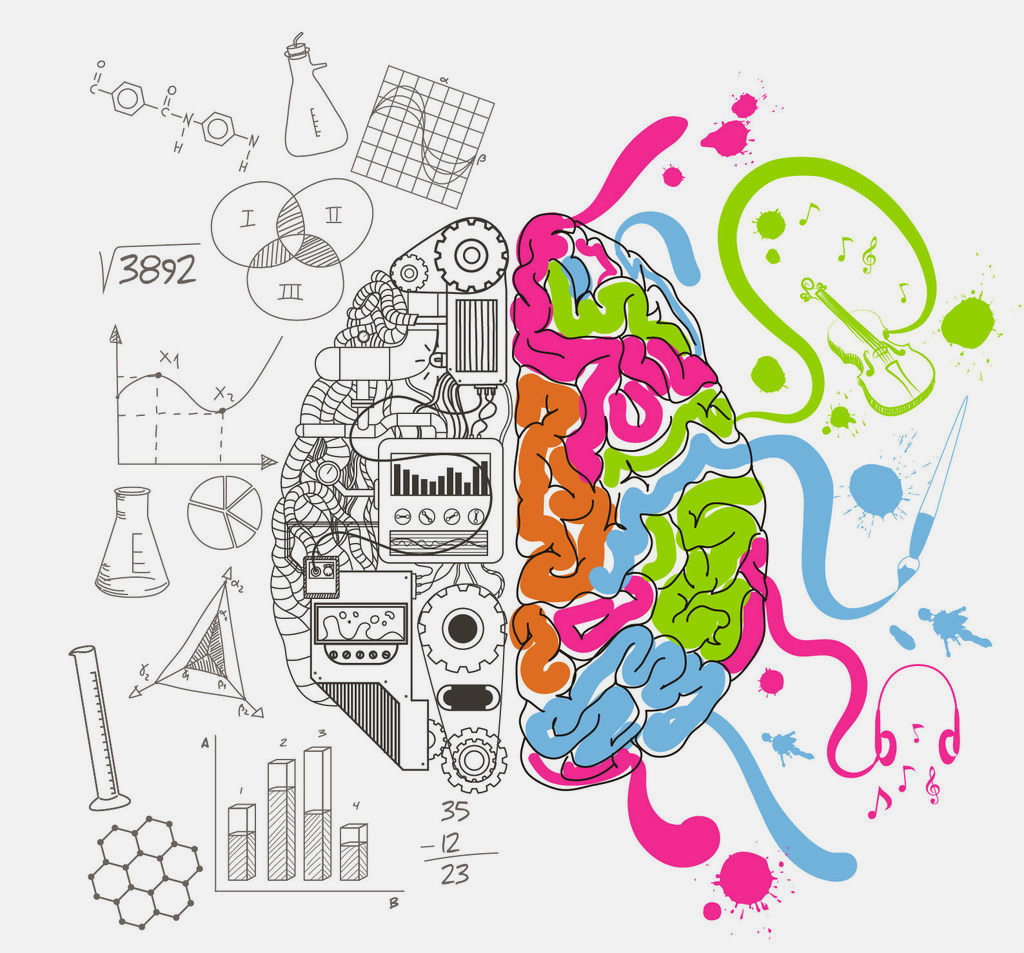

Representative work
Neural Computation, 2016
Journal of Neuroscience Methods, 2016
Neuroimage, 2011
IEEE Signal Processing Magazine, 2015
IEEE Transactions on PAMI, 2017
Theme #4: Translational and Computational Neuropsychiatry
Computational psychiatry is an emerging discipline that translates the knowledge of neuroscience to clinical applications. One active research area of computational psychiatry is to apply data-driven and machine learning approach to clinical data. Nowadays, concurrent transcranial current or magnetic stimulation (TCS/TMS) and electroencephalography (TCS/TMS-EEG) data have been used as a direct non-invasive method for measuring/modulating neocortical excitability, inhibition, and connectivity through neuroplasticity in healthy and neuropsychiatric population (e.g., chronic pain, depression, PTSD). One important and practical question in TCS/TMS is to optimize the stimulation parameters (e.g., stimulation site, intensity, orientation, etc.) and assess their effectiveness in an online fashion. Therefore, how to extract information in neurofeedback from EEG signals is the key. We will design innovative methods that integrate graph-theoretic measures, network connectivity, Granger causality analysis and reinforcement learning to resolve this challenging question.
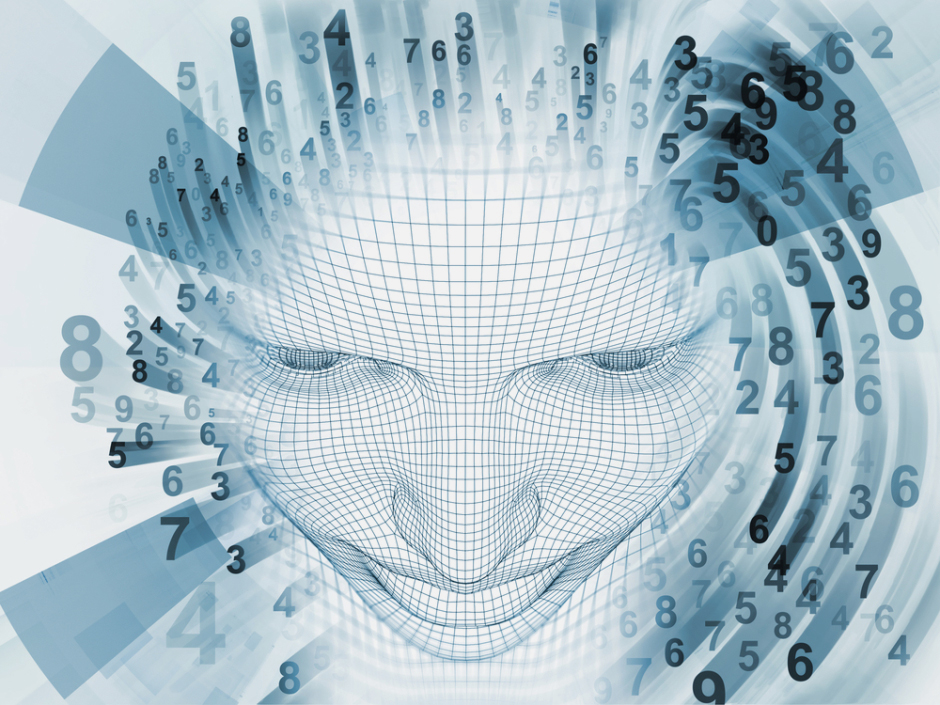